LLM Agent Frameworks 2025: Guide & Comparison

Ilias Ism
Apr 28, 2025
13 min read
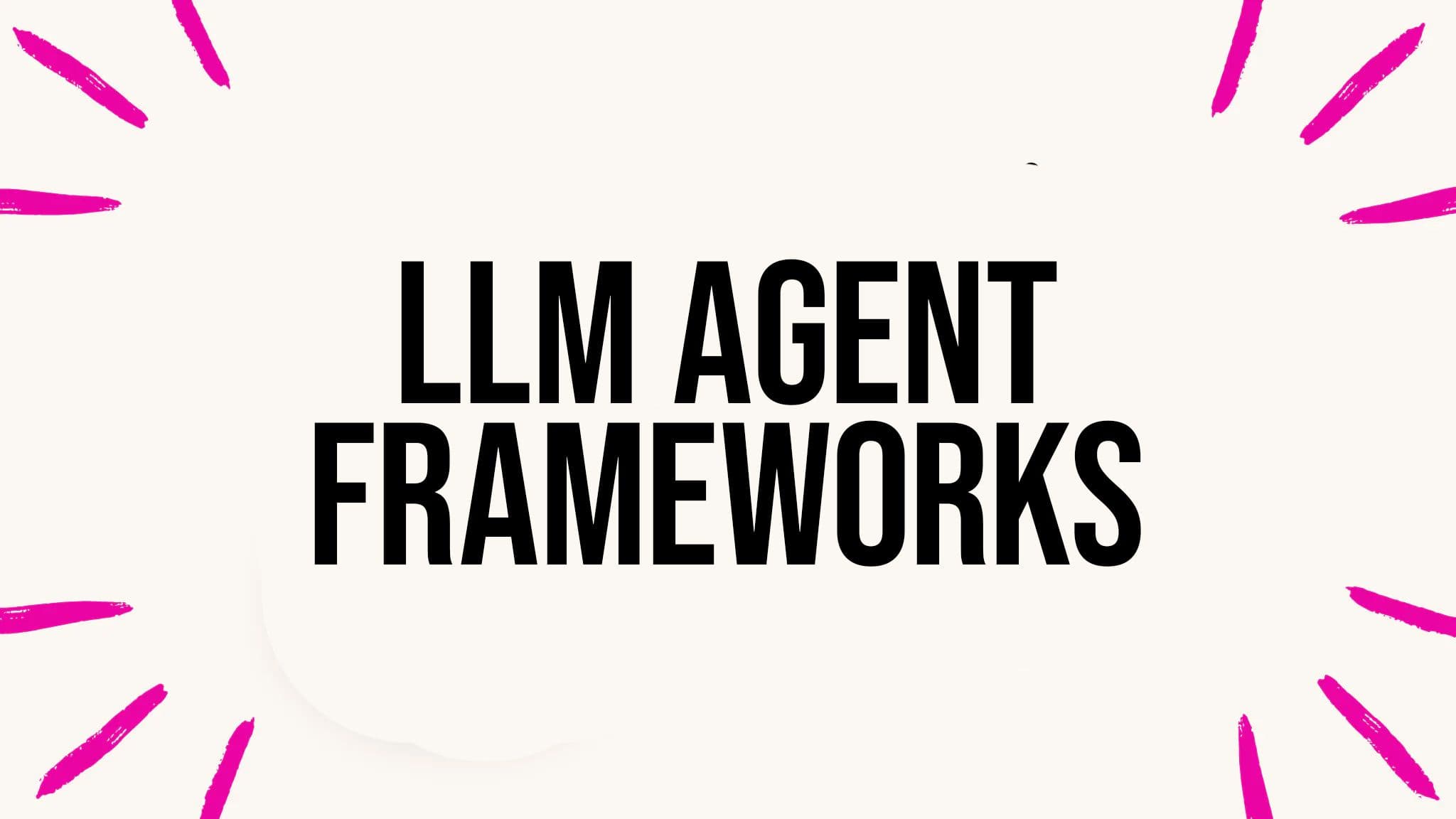
What are LLM agents, and how can you use them without complex code? This post explains agents and LLM agent frameworks: what they are, why they matter, their core parts, popular examples including multi-agent LLM frameworks, and how platforms like Chatbase help build chatbots using agentic systems.
A standard LLM can write and summarize but often lacks memory and can't interact with outside tools like databases.
LLMs also struggle with multi-step tasks requiring planning.
An LLM agent addresses these limits by using an LLM to understand its environment, make decisions, and take actions to achieve goals by planning steps, remembering context, using tools (like APIs or databases to check inventory or order status), and reasoning to adapt its actions.
Why Use LLM Agent Frameworks?
Building an agent from scratch is difficult.
Managing LLM communication, memory, tools, and plans requires significant code. LLM agent frameworks provide structure, offering pre-built components and standard ways to develop and manage agents.
Benefits: reusable structure, abstraction, easy integrations, logical orchestration, faster development.
Frameworks help models manage tasks, maintain context, and use external data.
Core Components of an LLM Agent Framework
Understanding the core components is key to grasping how any LLM agent framework operates. Most include:
- LLM core
- Planning module
- Memory module
- Tools/Tool Use
- Execution manager
These parts work cyclically: input -> plan -> action -> execute -> observe -> update memory -> repeat.
![[object Object]](/_next/image?url=https%3A%2F%2Fcdn.sanity.io%2Fimages%2Fi6kpkyc7%2Fprod-dataset%2F0ca86d8096b5970289233657e760daf362b5c43f-1024x1024.png&w=3840&q=75&dpl=dpl_Epb6xCnKKuE3vGbwbNx8kRiyhpKA)
Common LLM Agent Framework Examples & Comparison
The framework ecosystem includes several key players. Choosing depends on your technical skills and project needs.
![[object Object]](/_next/image?url=https%3A%2F%2Fcdn.sanity.io%2Fimages%2Fi6kpkyc7%2Fprod-dataset%2Fedc0d9fcf1c3282fd81c0168b5dde07d3a910c32-1460x1098.jpg&w=3840&q=75&dpl=dpl_Epb6xCnKKuE3vGbwbNx8kRiyhpKA)
Other Frameworks: MetaGPT, Google ADK, Botpress.
nimal LangChain Agent Code
main.py
1# Example: Basic LangChain Agent using ReAct2from langchain_openai import ChatOpenAI3from langchain.agents import tool, AgentExecutor, create_react_agent4from langchain_core.prompts import PromptTemplate56# Define a simple tool7@tool8def get_word_length(word: str) -> int:9 """Returns the length of a word."""10 return len(word)1112llm = ChatOpenAI(model="gpt-4o", temperature=0)13tools = [get_word_length]14template = """Answer the question using the available tools.1516Question: {input}"""17prompt = PromptTemplate.from_template(template)18agent = create_react_agent(llm, tools, prompt)19agent_executor = AgentExecutor(agent=agent, tools=tools, verbose=True)2021response = agent_executor.invoke({"input": "how many letters in the word 'serendipity'?"})22print(response["output"])
Multi-Agent Systems
Many frameworks support multi-agent systems.
Specialized agents work together on complex tasks. AutoGen and CrewAI focus specifically on enabling this collaboration.
What's New in LLM Agents (2025 Trends)
The field continues to evolve rapidly. Key trends emerging in 2025 include:
- Increased Reliability: Focus on making agent actions more predictable.
- Improved Tool Use: More sophisticated integration and selection of tools.
- Advanced Multi-Agent Collaboration: Better frameworks for coordinating teams of agents.
- Evaluation Frameworks: More robust methods for testing agent performance.
- Efficiency: Optimizations for reducing token usage and latency.
The Complexity of Code-Based Frameworks
Using frameworks like LangChain often requires:
- Coding Skills (Usually Python)
- Complex Setup
- Difficult Debugging
- Ongoing Maintenance
For businesses needing chatbots without dedicated development teams, these frameworks can be demanding.
Chatbase: A Simpler Way to Build AI Agents
![[object Object]](/_next/image?url=https%3A%2F%2Fcdn.sanity.io%2Fimages%2Fi6kpkyc7%2Fprod-dataset%2Fa7fed136eb7b529c68d4b7b745cc6aa1270bde1c-2630x1468.jpg&w=3840&q=75&dpl=dpl_Epb6xCnKKuE3vGbwbNx8kRiyhpKA)
Chatbase offers a different path. It's a no-code AI agent builder.
It makes agent capabilities accessible for applications like customer support without complex coding. Compare costs on our Pricing Page.
Chatbase uses agent principles in a user-friendly interface:
- LLM Core Choice: Select the underlying LLM.
- Simplified "Tools": Easily connect data sources (websites, documents) as knowledge tools.
- Built-in Memory: Handles conversation history for context.
- Goal-Oriented Setup: Define the bot's purpose via settings.
- Control Behavior: Guide responses and tasks via the interface.
![[object Object]](/_next/image?url=https%3A%2F%2Fcdn.sanity.io%2Fimages%2Fi6kpkyc7%2Fprod-dataset%2F8d17f11fbc4a5a6770b59aaa864f0c9ac147f029-2852x1386.png&w=3840&q=75&dpl=dpl_Epb6xCnKKuE3vGbwbNx8kRiyhpKA)
Building with Chatbase:
- Create Bot: Start a new project.
- Add Data Sources: Upload PDFs, link websites (e.g., FAQs). This is the bot's knowledge tool.
- Set Instructions: Write plain-language rules defining the bot's goal and behavior.
- Deploy & Refine: Add the bot to your site, test, and tweak as needed.
This approach gives you agent-like behavior (similar to Retrieval-Augmented Generation or RAG agents without the technical overhead.
The Future is Agent-Based and Accessible
LLM agents allow AI to solve problems actively. Frameworks provide structure for developers. However, this technology should be usable by more people. Platforms like Chatbase make it practical, offering intuitive tools for specific uses like customer service.
Understanding agents and frameworks is useful, whether coding or building visually. For a straightforward path to agent-based chatbots, platforms like Chatbase offer a clear solution.
Frequently Asked Questions (FAQs)
Q1: What's the cost associated with using LLM agent frameworks? A1: Open-source frameworks are free, but LLM API calls (pay-per-token) are the main cost. Complex agents increase costs. No-code platforms like Chatbase often bundle usage in subscriptions..
Q2: Are LLM agents ready for enterprise use? A2: Increasingly yes, with focus on reliability, control, and security. Simpler, task-specific agents are more mature than highly autonomous ones. Careful evaluation is essential.
Q3: Which framework is best for beginners? A3: For non-coders, a no-code platform like Chatbase. For Python developers new to agents, LangChain (complex but well-documented) or CrewAI (gentler multi-agent intro).
Q4: How much GPU power do I need to run LLM agents? A4: Usually none locally, as agents use cloud LLM APIs. Running open-source LLMs locally for agents does require significant GPU power.
Share this article: